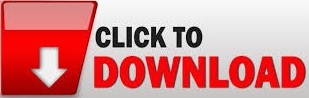
Notably, all three case studies include vast evidence of racial profiling, segregation, and discrimination within the hearts of supposedly impartial algorithms, indicating an exponentially bloated problem: administrative leadership’s refusal to correct course and redistribute resources as the welfare gap expands in American society. Based on a flawed premise and misrepresentation of variables, this system indirectly punished the poor for accessing public services. The final case study is the predictive risk model for child abuse from Allegheny County, Pennsylvania. Profiling turns into policing, eroding the illusion of impartial aid.

The second case study is the coordinated entry housing system in Los Angeles, where an algorithm scores neediness, hiding results from recipients but not from the police officers who hunt them. Eubanks connects its failures with overarching societal mechanisms that use discriminatory profiling on the impoverished. The first case study is the automated welfare eligibility system in Indiana. Eubanks argues that the digital entrapments of the modern welfare state are just a digital version of these rickety structures, making profit from poverty in a similar manner.

Critically lauded on publication, Automating Equality won the 2019 Lillian Smith Book Award, the 2018 McGannon Center Book Prize, and was shortlisted for the Goddard Riverside Stephan Russo Book Prize for Social Justice.Įubanks uses the history of the poorhouse-a way to punish and contain the impoverished in the 19th and early 20th centuries-as a metaphor for modern automated data and technological systems.
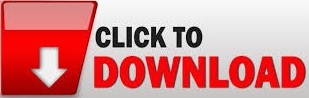